Use of Generative AI to Generate Synthetic Data in the Construction Industry

Abstract
ObraLink is exploring the use of generative artificial intelligence to address the lack of specific datasets in construction. This technology enables the creation of realistic images of construction sites, facilitating the training of computer vision models without relying solely on real data. This approach aims to enhance the precision and robustness of analytical algorithms in the industry.
Computer vision is a technology increasingly applied across various industries. Autonomous driving, surveillance, and infrastructure monitoring are just some examples of applications that—thanks to advances in hardware and deep learning algorithms—have integrated computer vision into their processes.
Although the construction sector has been slow to digitize, it has begun leveraging image analysis technologies for its tasks. Machinery tracking, safety equipment detection, and productivity measurement are some concrete applications of these technologies.
However, one of the biggest challenges in developing AI solutions for construction is the limited availability of specific datasets. While large public datasets like COCO, ImageNet, and PASCAL VOC exist, they mainly contain general-purpose images rather than construction-specific scenes.
At ObraLink, we already have construction site data captured by our Cibots. Every 20 minutes, these devices capture RGB images (color) and infrared images (to measure concrete temperature) from different sections of the construction site. Additionally, the Cibots continuously collect temperature and humidity data.
A viable alternative to address this issue is synthetic datasets. By generating artificial yet realistic data, we gain better control over environmental conditions, object diversity, and annotation precision, accelerating the development of reliable models for analysis.
How does this work?

Original polygon compared to the desired image
The process of designing these generative AI models (or GenAI in English) is the reverse of the traditional annotation process. In this case, we aim to move from a polygon or area to a realistic image of the construction site.
Example:

Original polygon compared to the image generated by the model
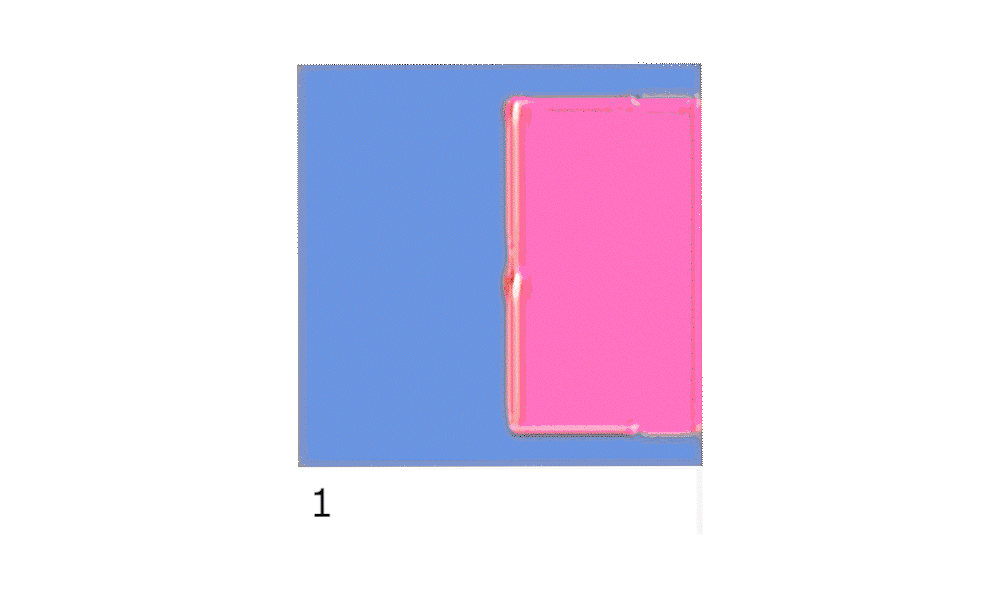
Iterations of the model
After selecting the base model, we trained it with around 2,000 images from different projects over a total training period of five hours. This resulted in the following output:
Despite the short training time, we can see that the model is capable of generating images very similar to the originals. The AI successfully represents recognizable elements of a construction site, such as scaffolding and railings, indicating that it has learned and captured the general patterns of the scene.
This project allowed us to conclude that artificial intelligence is a viable tool for generating synthetic datasets in the construction field.
If you want to learn more about the applications of artificial intelligence in construction, contact us here.